Hey Siri, naming your detector might just improve your data: how anthropomorphizing technology can help keep citizen scientists engaged
Paper Title: Anthropomorphism and motivating participation in citizen science projects
Author(s) and Year: Zoey Rosen, Marilee Long, Bonne Ford, Eric A. Wendt, Michael Cheeseman, Casey Quinn, Christian L’Orange, John Volckens, and Jeffrey R. Pierce, 2024
Journal: JCOM Journal of Science Communication (open access)
TL;DR: Citizen science can be an effective way to collect large datasets over long periods of time. However, maintaining participation in citizen science projects can be challenging as participants have different motivations than traditional scientists. This study found that anthropomorphizing the technology used in the scientific endeavor can slightly improve the motivation of citizen scientists, as it can help participants feel more connected to the work.
Why I chose this paper: I have been curious about citizen science projects for a while, both as a way to increase public understanding of science as well as an effective way to gather larger amounts of scientific data, and I was interested to learn about ideas to maintain participant involvement in these projects. As a scientist, I can personally attest to practicing some anthropomorphizing techniques (particularly naming and ascribing intent) on particularly troublesome projects to reduce frustration in setbacks or technological hurdles, so it was interesting to learn why these techniques can be helpful.
Fold-It is an online puzzle video game that was launched in 2008 and soon gained hundreds of thousands of players. Only Fold-It wasn’t designed by a technology startup; instead, it was a project launched by scientists studying protein structure who realized that the dataset was larger than they could tackle by themselves. Through Fold-It, these researchers were able to enlist the help of everyday citizens to help understand a pressing scientific question while simultaneously engaging the public in the scientific process.
The Background
Over the past decades, citizen science projects like Fold-It have become an increasingly popular way for scientists to collect and/or analyze large datasets that would be onerous to do by themselves, especially across large time or spatial scales. However, while participants are generally volunteers interested in the scientific project, motivation can wane over time. This can be especially problematic when research projects use complicated technology to aid their study; not only does the technology require more training for the citizen scientists, but some individuals may feel less agency, understanding, or connection to the endeavor and be less intrinsically motivated as a result. Looking at these efforts through the lens of human-robot interaction (HRI), Rosen et al. aimed to understand how connecting to research technology in human-like ways (e.g. naming, ascribing intent or emotions, etc.) might impact participant motivation in their corresponding citizen science project.
The Methods
The SEEK (sociality, effectance, and elicited agent knowledge) framework is an established method in the HRI field to understand how people interact and understand robotic technology. Specifically, individuals may anthropomorphize a robot in order to feel a connection to it (sociality), to understand or reduce uncertainty in its complicated processes (effectance), and to process an unfamiliar behavior through their own perspective (elicited agent knowledge). The SEEK model can be extended to a wide variety of fields, from animal behavior to extreme weather to robots. In this study, the researchers looked to map the SEEK framework onto the three most common reasons people give for participating and remaining active in citizen science projects: building community amongst like-minded individuals (sociality), feeling competent enough to perform the required tasks (effectance), and contributing to scientific knowledge (elicited agent knowledge).
Rosen et al. recruited students in a summer program to join the Citizen-Enabled Aerosol Measurements for Satellites (CEAMS), in which citizen scientists utilized a robotic sampler (Fig 1) to measure local air quality in regions across the country. The students were given training to encourage them to credit human-like qualities (such as naming, pronouns, and ascribing intention) to the sampler, and the sampler itself was programmed to behave in more human-like ways, including a “wake up” sequence. Over the course of the study – including before the anthropomorphizing training – the researchers gave the students a questionnaire that measured both the SEEK factors and top reasons for participating in citizen science. They also asked the students to keep a journal of their work, which was analyzed to determine the amount of anthropomorphic language the participants used to describe the sampler.
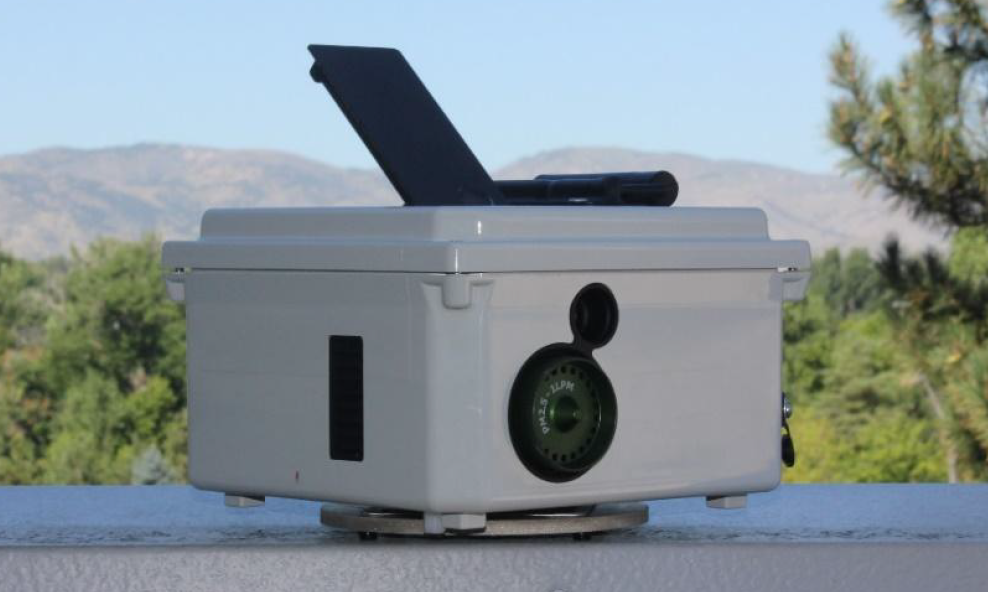
The Results
Overall, the scientists found that training members to anthropomorphize their robots increased the human-coded language the trainees assigned to their sampler. For example, a student who received the training was more likely to name or gender their sampler, to attribute intention or feeling (“the sampler prefers…”), or to generally give lifelike qualities to it.
The researchers also determined that they were able to map the SEEK model onto key intrinsic rewards for citizen scientists. So, in general, individuals who felt more socially connected to their sampler also reported that building community was a more important factor in their work. Similarly, those who understood complicated sampler processes in anthropomorphic terms (“the robot woke up and proceeded to look for the proper direction…”) usually reported feeling more competent. On average, participants also reported feeling more competent and valuing their contribution to science as the study proceeded. Only “building community” was reported to decrease, although the researchers note that this was likely a byproduct of COVID precautions rather than any specific finding of this study.
Because the research study needed to be completed on a reasonable timescale with a consistent number of participants, the researchers were unable to comment on the long-term commitment of the citizen scientists. However, by quantifying the effect of anthropomorphizing training and establishing the connection between the SEEK model and top motivations for partaking in citizen science projects, they have added a useful framework to understand how to improve public commitment in these large endeavors, and how scientists might be able to more clearly communicate complicated scientific processes in general.
The Impact
Public trust in science has declined in recent years, most often amongst individuals who have little to no interaction or understanding of the scientific process. Citizen science offers an avenue to bridge the gap between the public and scientists, but maintaining interest in projects that can take months or even years is difficult even for scientists, let alone citizens. This can be especially true when contributors are asked to work with more and more complicated technology. This study offers promise on that front by extending the SEEK model to citizen science projects to help researchers understand participant motivation. Overall, it shows that anthropomorphizing robotic tech can help people understand these often-sophisticated processes.
Written by Clark Hickman
Edited by Julianna Goenaga and Madeline Fisher
Featured image credit: TheDigitalArtist from Pixabay